Jan 2019 | Niranjan Pedanekar | 1124 words | 5-min read
What does a data scientist do all day? Niranjan Pedanekar is a Principal Scientist at Tata Consultancy Services (TCS) Research. Here he gives an insight into a typical day in the office:
I get up around 7.30am and head straight into the office. I’m in charge of a group called Area 66. All of us are data scientists and we all have varying levels of experience.
I put together this group around two years ago because I was really interested in entertainment and how humans engage with it. I’m also a playwright, actor and director, so I wrestle with these same questions in my work there as well.
What my team does falls somewhere between academic research and industry work and it sits at the intersection between entertainment, data science and behavioral science.
We’re currently looking at the applications of AI for the entertainment, media and advertising industries. We write algorithms which enable us to annotate media in various ways automatically.
Let’s take movies, for example. We’re trying to build an algorithm which can recognize the different emotional intensities within a movie. So, an action sequence is high intensity, but a scene where people are sitting in a meeting room and talking is low intensity. The data we use for this is things like the color palette, the music and the speed of the action in a sequence.
Bringing AI to the movies
You might be wondering why we do this. Well, there are various applications for it. One is the placement of adverts, which can sometimes jar with the action of the movie. You might have a really serious scene, followed by an advert full of people dancing, and then you’re back to the somber movie again.
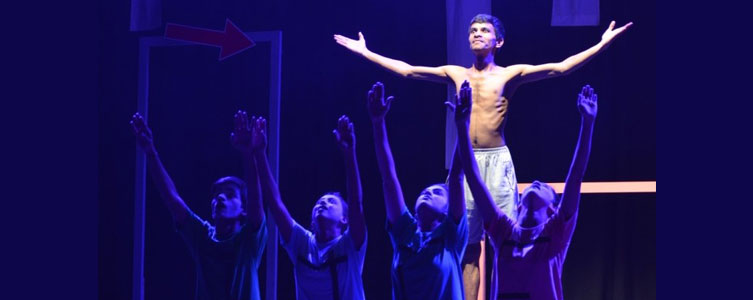
Our technology would allow adverts to match the mood of the movie – an advert for a drink after a scene set in the desert or an advert for sneakers after a scene where people are running.
Also, we’re looking at how to annotate movies based on the setting. So, it would be able to distinguish between a chase scene in a forest and a tranquil scene on the beach. This could help Netflix, Amazon, and other streaming services, give their viewers a more personalized experience.
Let’s say you’re a Matrix fan, and you’ve seen the movies countless times, you only want to see the action scenes. This would allow you to do that.
There are societal benefits too. In the future, and this is on our agenda, we could annotate movies for violence or things unsuitable for children. If a family is watching a movie they are all enjoying, but if there is one scene that might upset the youngest viewer, the algorithm could spot that. A lot of our day is spent looking at such data and trying to train algorithms to learn from it.
Keeping up with the data set
We also need to read a lot about what is happening elsewhere. Most of my day goes into reading the papers others are writing about their research. As a data scientist, you don’t want to be left behind. You also need to understand what’s going on in the world, so I read newspapers a lot too.
Good research happens at the intersection of fields, so I might also read psychology or behavioral science papers, as well as AI ones. There are a lot of behavioral aspects in advertising, so if you want to introduce AI into it, you need to understand how people react to things. We also write our own research. Our group writes between five and 10 papers a year and attends as many conferences.
We meet on a weekly basis and exchange ideas, but informally we interact almost daily over lunch or just casually passing by someone’s desk. Sometimes one of us might really want to do something that sounds really interesting, but it may not immediately pay dividends. So that’s my job as the manager of the group, I have to take a call on what research we should pursue.
The importance of creativity
I usually finish work at six o’clock and, most of the time, I head straight to a rehearsal, which might go on until midnight.
My day is a continuum of entertainment, AI and art coming together. I almost don’t distinguish between the two. If I think of a play idea and I need to jot down the outline, I will do that right away. And if I’m working on a production, and an idea strikes me about my AI work, I get on it.
You need that creativity in data science. When you are stuck with a problem, you have to find various ways of getting out of that problem. It’s the same when you are directing plays, you need to look at whatever is written and imagine five different ways in which it could be interpreted.
Being a “good” data scientist
You need to have a good grasp of the problem at hand and understand what your work will produce and how it will help. Say, for example, I’m working on an algorithm that can detect cancer from the scans of patients, I need to understand what the accuracy of the algorithm actually means. I can write a paper saying that I improved the results from 95% accuracy to 96.3%, but what does that really mean in terms of saving a life? Is that another two or three hundred lives saved?
It’s really important to be able to understand numbers and trends and how things affect each other, rather than just being sucked into the algorithms. You need to make sense out of them. The new wave of algorithms, which is the deep learning, often don’t come up with answers that can be explained. So you need to understand whether that is OK for you.
That’s part of the ethical considerations about data science as well. In some ways you can compare data science to fire. At some point, someone invented fire which can be used for really good things, or really bad things. The same can be said of AI, it can be used for cancer diagnosis or it can be used for weaponry.
Some might say they’re not going to worry about those things because it’s the science they care about. But someone somewhere has to worry. Even someone like Elon Musk, who is a big advocate for AI, also warns people about the bad effects that it might have in the future. It’s about trying to put checks and balances in on how to use it for a good cause.
Niranjan Pedanekar is a Principal Scientist at TCS Research, Pune. This post was previously published on the Digital Empowers blog.